Chapter
2. Finding genes for common diseases
Introduction
Finding genes for common diseases
In 2007, a consortium of fifty British research groups collectively known as the Wellcome Trust Case Control Consortium (WTCCC) investigated the genetic basis of seven common human diseases—rheumatoid arthritis, hypertension, Crohn's disease (the most common form of inflammatory bowel disease), coronary artery disease, bipolar disorder (manic depression), type-1 diabetes, and type-2 diabetes—in the largest genome-wide association study (GWAS) performed at that time1. Your textbook discusses this particular GWAS in Section 19.6. Why has the WTCCC GWAS received so much attention? The WTCCC study was groundbreaking in many ways. It not only confirmed the involvement of some previously reported genes, but it also identified several additional genes that affect susceptibility to common diseases. In many cases, it doubled the number of genes associated with these disorders. Moreover, it established a successful and rigorous approach to large-scale GWASs of this type and demonstrated that a variety of diseases or traits can be analyzed at the same time using a shared set of control subjects. The success of the WTCCC study also motivated researchers to pursue GWASs on an even larger scale. In response, a flurry of studies that followed provided novel insights into the causes of many forms of human disease. In some cases, the findings propelled the development of innovative disease treatments. Many questions remain to be answered about how the genes identified contribute to their associated diseases, however.
1. Genome-wide association study of 14,000 cases of seven common diseases and 3,000 shared controls. Nature 447, 661–678 (2007). doi: nature05911
Basis of the Global Association Study
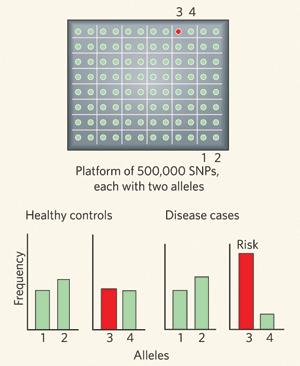
Figure 1: Genome-wide association scan.
Figure 1 Source: Figure 1 from A. M. Bowcock. Genomics: Guilt by association. Nature 447, 645-646 (7 June 2007). doi: 10.1038/447645a http://www.nature.com/nature/journal/v447/n7145/fig_tab/447645a_F1.html
In one GWAS, the WTCCC investigators examined genetic variation at 500,000 different positions within the genomes of 17,000 individuals living in the United Kingdom (Figure 1). This statistical approach compares the frequencies of genetic variation in individuals with a given disease and in control individuals from the same population. There are approximately ten million such changes (known as single nucleotide polymorphisms, or SNPs) located at particular positions scattered throughout the human genome where variation is found in at least 10% of the population. In a GWAS, researchers examine the relationship between every DNA position and a particular trait (such as diabetes) using the signal from each position as an indicator for the DNA sequence that surrounds it. A strong “association'” between a DNA position and a particular disease or trait marks the general location of the associated genetic alteration, even if the associated SNP itself is not directly responsible for the disease (discussed in your textbook in Section 19.6).
The concept of drawing an association between biological traits and disease is not new2, but the overall scope and scale of the WTCCC in its application of this concept was unparalleled. The following factors were crucial to the success of this study and to keeping costs within reasonable limits: access to DNA samples from large numbers of unrelated patients from the United Kingdom; knowledge of the complete sequence of the human genome; the availability of a vast number of SNPs3; the completed HapMap project4, which provided information about the genetic relatedness of SNPs; and the availability of high-throughput technologies, which allowed the simultaneous analysis of 500,000 genetic markers that collectively represented most of the common variation in the human genome.
2. Billington, B. P. Gastric cancer; relationships between ABO blood-groups, site, and epidemiology. Lancet 271, 859–862 (1956).
3. Carlson, C. S. et al. Selecting a maximally informative set of single-nucleotide polymorphisms for association analyses using linkage disequilibrium. American Journal of Human Genetics 74, 106–120 (2004). doi: 10.1086/381000
4. A haplotype map of the human genome. Nature 437, 1299–1320 (2005). doi: 10.1038/nature04226
WTCCC Findings and Follow-up Studies
Among the seven common diseases, the WTCCC study yielded statistically significant evidence for genetic associations with twelve previously identified genomic regions and with twelve new genomic regions (Figure 2). Although the WTCCC report provided an initial glimpse of these associations, independent studies by other research groups subsequently confirmed all but one of the most significant regions identified by the WTCCC through replication studies5, 6, 7. Furthermore, follow-up studies of some of the other regions originally identified by the WTCCC as showing less statistically significant disease associations demonstrated that those regions are, in fact, associated with significant genetic risk8. The results of these follow-up studies are a shining example of the value and importance of independent verification of experimental outcomes.
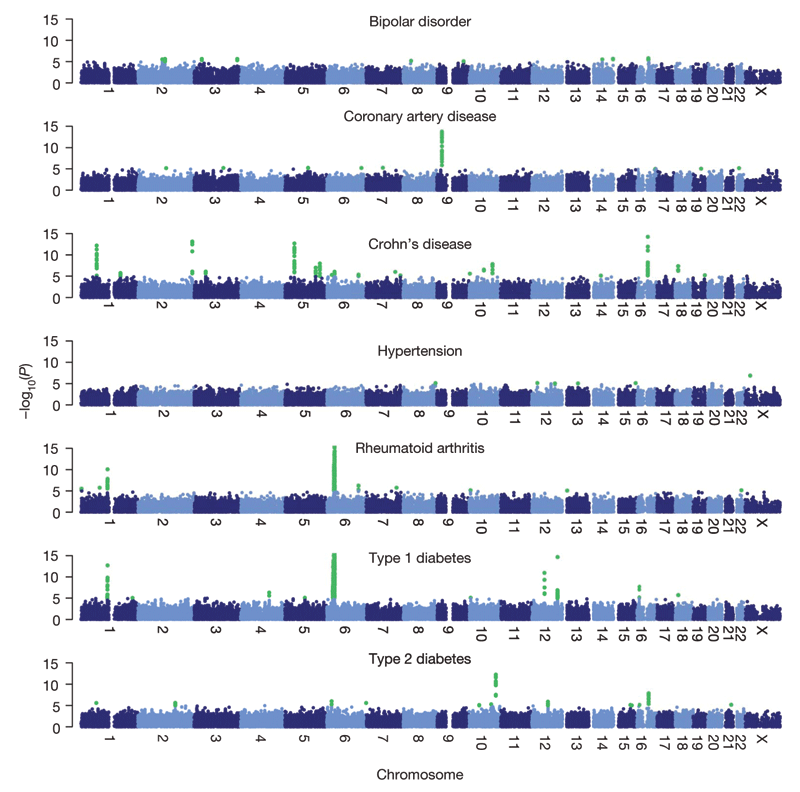
Figure 2: Results of WTCCC genome-wide association scan for seven diseases.
Figure 4 from Wellcome Trust Case Control Consortium. Genome-wide association study of 14,000 cases of seven common diseases and 3,000 shared controls. Nature 447, 661-678 (7 June 2007). doi: 10.1038/nature05911 http://www.nature.com/nature/journal/v447/n7145/fig_tab/nature05911_F4.html
The WTCCC data are publicly available and have proven to be an invaluable resource to other groups and consortia engaged in similar studies to identify genetic markers linked to these and other diseases. Even larger studies have been performed since the WTCCC study, in some cases involving more than 100,000 individuals9, 10. One is a study of body mass with approximately 2.8 million SNPs, revealing thirty-two loci, some mapping to genes encoding regulators of appetite, energy balance, or levels of secreted insulin (Figure 3)11. As of April 2015, nearly six hundred GWASs have been performed and more than two thousand associations between genetic regions and specific traits have been reported12 (updated at (http://www.ebi.ac.uk/gwas/home).
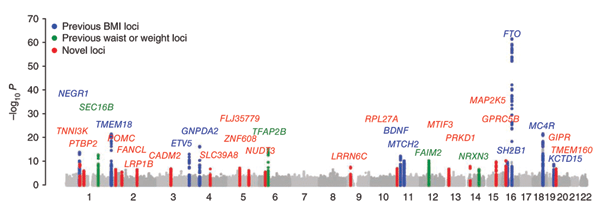
Figure 3: Genome-wide SNP association results for body mass index.
Figure 1 (panel a only) from E. K. Speliotes et al. Association analyses of 249,796 individuals reveal 18 new loci associated with body mass index. Nature Genetics 42, 937-948 (2010). doi: 10.1038/ng.686 http://www.nature.com/ng/journal/v42/n11/fig_tab/ng.686_F1.html
5. Todd, J. A. et al. Robust associations of four new chromosome regions from genome-wide analyses of type 1 diabetes. Nature Genetics 39, 857-864 (2007). doi: 10.1038/ng2068
6. Zeggini, E. et al. Replication of genome-wide association signals in UK samples reveals risk loci for type 2 diabetes. Science 316, 1336-1341 (2007). doi: 10.1126/science.1142364
7. Saxena, R. et al. Genome-wide association analysis identifies loci for type 2 diabetes and triglyceride levels. Science 316, 1331-1336 (2007). doi: 10.1126/science.1142358
8. D'Addabbo, A. et al. Discovering genetic variants in Crohn's disease by exploring genomic regions enriched of weak association signals. Digestive and Liver Disease [Epub ahead of print] (2011). doi: 10.1016/j.dld.2011.02.010
9. Teslovich, T. M. et al. Biological, clinical and population relevance of 95 loci for blood lipids. Nature 466, 707-713 (2010). doi: 10.1038/nature09270
10. Lango Allen, H. et al. Hundreds of variants clustered in genomic loci and biological pathways affect human height. Nature 467, 832-838 (2010). doi: 10.1038/nature09410
11. Speliotes, E. K. et al. Association analyses of 249,796 individuals reveal 18 new loci associated with body mass index. Nature Genetics 42, 937-948 (2010). doi: 10.1038/ng.686
12. Manolio, T. A. Genomewide association studies and assessment of the risk of disease. New England Journal of Medicine 363, 166-176 (2010). doi: 10.1056/NEJMra0905980
The Next Step Following a GWAS
After a particular disease or trait has been associated with a particular marker SNP, what happens? The next step is to move beyond the associated marker SNP to study the exact nature of the variant that is responsible for the disease or trait. At what types of locations within the genome do the responsible variants typically occur? Overall, it seems that the variants leading to common diseases are diverse: only approximately 10% are located within genes where they alter coding sequences13, whereas 45% are located within the noncoding sequences of genes and approximately 45% are located between genes; some variants even lie within gene deserts14, which are chromosomal regions that contain few or no genes. The approximately 90% of variants that are located outside of gene coding sequences are expected to be involved in gene regulation, but identifying the true variants and understanding their biological role remain formidable challenges.
13. Hindorff, L. A. et al. Potential etiologic and functional implications of genome-wide association loci for human diseases and traits. Proceedings of the National Academy of Sciences of the United States of America 106, 9362-9367 (2009). doi: 10.1073/pnas.0903103106
14. Libioulle, C. et al. Novel Crohn disease locus identified by genome-wide association maps to a gene desert on 5p13.1 and modulates expression of PTGER4. PLoS Genetics 3, e58 (2007). doi: 10.1371/journal.pgen.0030058
The First GWAS
The first study demonstrating that a GWAS might be a feasible approach for identifying genes responsible for a common disease was focused on age-related macular degeneration (AMD), which is the third leading cause of blindness. The results of this pioneering study showed an association between AMD and the complement factor H (CFH) gene15. The results were spectacularly successful given the small number of samples (ninety-six AMD patient samples and fifty control samples), and they set the stage for the GWAS studies that have followed. Approximately 40% of the genetic variance of AMD can be explained by common variants at five SNPs16. For each SNP, the risk of disease is two- to three-fold higher than the risk for a person without one of the variants16. The results of these studies also provided evidence that a pathway that regulates inflammation is central to AMD-associated pathogenesis.
15. Klein, R. J. et al. Complement factor H polymorphism in age-related macular degeneration. Science 308, 385-389 (2005). doi: 10.1126/science.1109557
16. Sobrin, L. et al. Genetic profile for five common variants associated with age-related macular degeneration in densely affected families: A novel analytic approach. European Journal of Human Genetics 18, 496-501 (2010). doi: 10.1038/ejhg.2009.185
Crohn's Disease
In the WTCCC study, one of the newly identified susceptibility genes for the inflammatory bowel disease Crohn's disease was autophagy-related protein 16-like 1 (ATG16L1). This finding was of particular interest because it controls the spread of intracellular pathogens by a process involved in cellular self-digestion called autophagy. This exciting and unexpected discovery firmly established a role for gut bacteria in the pathogenesis of Crohn's disease17. Since then, more than seventy risk variants for Crohn's disease have been identified18. Figure 4 describes a model for inflammatory bowel disease pathways based on GWASs of both Crohn's disease and the related disease ulcerative colitis.
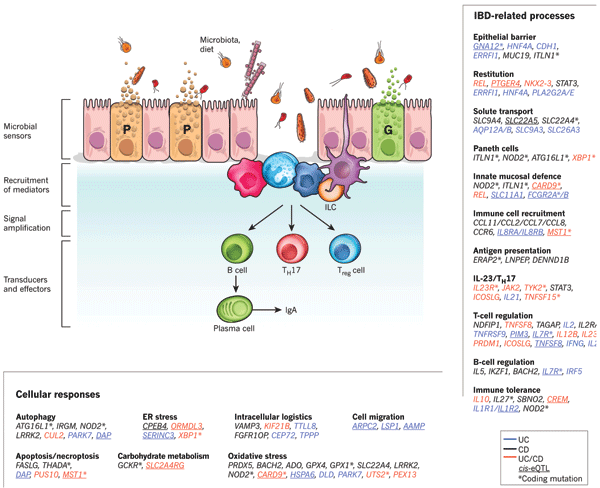
Figure 4: A model for IBD pathways based on GWAS.
Figure 2 from Genetics and pathogenesis of inflammatory bowel disease. Nature 474, 307-317 (16 June 2011). doi: 10.1038/nature10209http://www.nature.com/nature/journal/v474/n7351/fig_tab/nature10209_F2.html
17. Budarf, M. L. et al. GWA studies: Rewriting the story of IBD. Trends in Genetics 25, 137-146 (2009). doi: 10.1016/j.tig.2009.01.001
18. Franke, A. et al. Genome-wide meta-analysis increases to 71 the number of confirmed Crohn's disease susceptibility loci. Nature Genetics 42, 1118-1125 (2010). doi:10.1038/ng.717
Benefits of GWAS
Why are GWAS approaches so valuable? A GWAS can implicate previously unsuspected pathways that are altered during disease, which can lead to the development of new therapies. For example, the discovery that inflammation plays a role in AMD is now being used to develop novel forms of treatment. In addition, knowledge of genetic variation within two genes associated with type-2 diabetes19 (called TCF7L2 and KCNJ11) in patients is being used to look at the response to certain drugs for diabetes, and a gene associated with psoriasis20 (called IL12B) has highlighted an altered cellular pathway that is currently being targeted with new therapies. In an association study of plasma lipid levels where 95 loci were identified9, one of them—HMGCR—is the target of statins, which are taken to lower low-density lipoprotein (LDL) levels and reduce the risk of myocardial infarction. Notably, some common diseases share certain risk factors, so drugs developed for one disease might effectively target another.
19. Pearson, E. R. Pharmacogenetics and future strategies in treating hyperglycaemia in diabetes. Frontiers in Bioscience 14, 4348-4362 (2009).
20. Krueger, G. G. et al. A human interleukin-12/23 monoclonal antibody for the treatment of psoriasis. New England Journal of Medicine 356, 580-592 (2007). doi: 10.1056/NEJMoa062382
9. Teslovich, T. M. et al. Biological, clinical and population relevance of 95 loci for blood lipids. Nature 466, 707-713 (2010). doi: 10.1038/nature09270
Remaining Questions
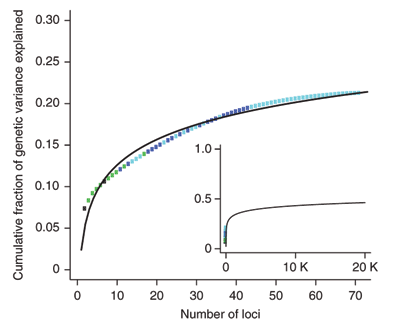
Figure 5: Cumulative fraction of genetic variance explained by seventy-one Crohn's disease risk loci.
Figure 2 from Genome-wide meta-analysis increases to 71 the number of confirmed Crohn's disease susceptibility loci. Nature Genetics 42, 1118-1125 (2010). doi: 10.1038/ng.717 http://www.nature.com/ng/journal/v42/n12/fig_tab/ng.717_F2.html
Despite the magnitude and wealth of information provided by both the WTCCC study1 and other recent studies that were even larger in scope, many questions remain about the genetic basis of common diseases. For example, the overall increase in disease risk conferred by the genetic factors identified in the WTCCC study1 and many others is low: approximately 1.2 to 1.5 times (1.33 is the median)13. GWASs also fail to account for all the heritability for any disease or trait. In the case of Crohn's disease, less than 25% of the genetic variation thought to account for its development has been identified to date18 (Figure 4), and in the case of human height, the 180 associated loci that have been identified account for only 10% of its phenotypic variation10. Recent statistical studies suggest, however, that within many GWAS data sets, there are additional signals to uncover that could account for some of the remaining the heritability of traits. For example, scientists estimate that GWASs can uncover at least 33% of the heritability for schizophrenia and that some of this heritability will be shared with bipolar disorder21. In addition, scientists estimate that approximately 20% of heritable variation in height can be discovered with GWASs10.
1. Genome-wide association study of 14,000 cases of seven common diseases and 3,000 shared controls. Nature 447, 661–678 (2007). doi: nature05911
13. Hindorff, L. A. et al. Potential etiologic and functional implications of genome-wide association loci for human diseases and traits. Proceedings of the National Academy of Sciences of the United States of America 106, 9362-9367 (2009). doi: 10.1073/pnas.0903103106
18. Franke, A. et al. Genome-wide meta-analysis increases to 71 the number of confirmed Crohn's disease susceptibility loci. Nature Genetics 42, 1118-1125 (2010). doi:10.1038/ng.717
10. Lango Allen, H. et al. Hundreds of variants clustered in genomic loci and biological pathways affect human height. Nature 467, 832-838 (2010). doi: 10.1038/nature09410
21. Purcell, S. M. et al. Common polygenic variation contributes to risk of schizophrenia and bipolar disorder. Nature 460, 748-752 (2009). doi: 10.1038/nature08185
How GWASs Can Translate into Personalized Medicine
How will GWAS findings affect the future of medicine? We are entering era of personalized medicine in which an individual's genetic makeup will eventually determine how his or her therapy is tailored. Therefore, an increased understanding of the genetic basis of common diseases is becoming increasingly critical. It is imperative that we understand how genes predispose individuals to diseases, how disease risk is affected when genes interact with one another, and how rare variants that are difficult to detect using current methods contribute to diseases.
What are some other pressing issues associated with translating GWAS data into personalized medicine? We'd like to know whether different patients can be grouped into subpopulations based on their genetic risk factors, and we'd like to understand the role the environment plays in triggering disease. The Genes, Environment, and Health Initiative of the National Institutes of Health (NIH) (http://www.niehs.nih.gov/health/topics/science/gene-env/index.cfm) is focused on the development of tools for environmental assessment, which may provide answers to some of these remaining questions. Ultimately, comprehensive answers will require much larger patient populations, detailed clinical databases, and sophisticated environmental assessments to ultimately translate these findings into scientifically sound medical practice.
Summary
Many common human diseases and traits are due in part to common genetic variants. Currently, GWASs are being used to identify these genetic variants. This powerful approach relies on the investigation of large populations of diseased individuals and control individuals from the same population with expansive numbers of DNA markers to identify most of the common genetic variants in the human genome. Over the last few years, more than six hundred GWASs have been performed, leading to the identification of more than seven hundred genetic regions associated with a wide range of human diseases. Despite these successes and the identification of novel pathways for drug development, less than 30% of the genetic contribution to disease has been explained to date. Over the next few years, GWASs are likely to be replaced by complete genomic sequencing, which should help explain the remaining heritability. Progress in identifying genetic risk factors for common diseases and traits is expected to continue at a rapid pace and will undoubtedly lead to exciting new discoveries that will positively affect disease prevention and treatment.
References
1. Genome-wide association study of 14,000 cases of seven common diseases and 3,000 shared controls. Nature 447, 661–678 (2007). doi: nature05911
2. Billington, B. P. Gastric cancer; relationships between ABO blood-groups, site, and epidemiology. Lancet 271, 859–862 (1956).
3. Carlson, C. S. et al. Selecting a maximally informative set of single-nucleotide polymorphisms for association analyses using linkage disequilibrium. American Journal of Human Genetics 74, 106–120 (2004). doi: 10.1086/381000
4. A haplotype map of the human genome. Nature 437, 1299–1320 (2005). doi: 10.1038/nature04226
5. Todd, J. A. et al. Robust associations of four new chromosome regions from genome-wide analyses of type 1 diabetes. Nature Genetics 39, 857-864 (2007). doi: 10.1038/ng2068
6. Zeggini, E. et al. Replication of genome-wide association signals in UK samples reveals risk loci for type 2 diabetes. Science 316, 1336-1341 (2007). doi: 10.1126/science.1142364
7. Saxena, R. et al. Genome-wide association analysis identifies loci for type 2 diabetes and triglyceride levels. Science 316, 1331-1336 (2007). doi: 10.1126/science.1142358
8. D'Addabbo, A. et al. Discovering genetic variants in Crohn's disease by exploring genomic regions enriched of weak association signals. Digestive and Liver Disease [Epub ahead of print] (2011). doi: 10.1016/j.dld.2011.02.010
9. Teslovich, T. M. et al. Biological, clinical and population relevance of 95 loci for blood lipids. Nature 466, 707-713 (2010). doi: 10.1038/nature09270
10. Lango Allen, H. et al. Hundreds of variants clustered in genomic loci and biological pathways affect human height. Nature 467, 832-838 (2010). doi: 10.1038/nature09410
11. Speliotes, E. K. et al. Association analyses of 249,796 individuals reveal 18 new loci associated with body mass index. Nature Genetics 42, 937-948 (2010). doi: 10.1038/ng.686
12. Manolio, T. A. Genomewide association studies and assessment of the risk of disease. New England Journal of Medicine 363, 166-176 (2010). doi: 10.1056/NEJMra0905980
13. Hindorff, L. A. et al. Potential etiologic and functional implications of genome-wide association loci for human diseases and traits. Proceedings of the National Academy of Sciences of the United States of America 106, 9362-9367 (2009). doi: 10.1073/pnas.0903103106
14. Libioulle, C. et al. Novel Crohn disease locus identified by genome-wide association maps to a gene desert on 5p13.1 and modulates expression of PTGER4. PLoS Genetics 3, e58 (2007). doi: 10.1371/journal.pgen.0030058
15. Klein, R. J. et al. Complement factor H polymorphism in age-related macular degeneration. Science 308, 385-389 (2005). doi: 10.1126/science.1109557
16. Sobrin, L. et al. Genetic profile for five common variants associated with age-related macular degeneration in densely affected families: A novel analytic approach. European Journal of Human Genetics 18, 496-501 (2010). doi: 10.1038/ejhg.2009.185
17. Budarf, M. L. et al. GWA studies: Rewriting the story of IBD. Trends in Genetics 25, 137-146 (2009). doi: 10.1016/j.tig.2009.01.001
18. Franke, A. et al. Genome-wide meta-analysis increases to 71 the number of confirmed Crohn's disease susceptibility loci. Nature Genetics 42, 1118-1125 (2010). doi:10.1038/ng.717
19. Pearson, E. R. Pharmacogenetics and future strategies in treating hyperglycaemia in diabetes. Frontiers in Bioscience 14, 4348-4362 (2009).
20. Krueger, G. G. et al. A human interleukin-12/23 monoclonal antibody for the treatment of psoriasis. New England Journal of Medicine 356, 580-592 (2007). doi: 10.1056/NEJMoa062382
21. Purcell, S. M. et al. Common polygenic variation contributes to risk of schizophrenia and bipolar disorder. Nature 460, 748-752 (2009). doi: 10.1038/nature08185
Quiz
Question
2.1
GCVs5wMeHDWBt6I2UAmZb0KQw6g5AAikAqA2oUI+qDo8beW+mhJeiO93DAnpgOP0TrFT0UfJMr/x/5qTJynRCw/+Nlo1e1aaYzA4qDnVTJn0/S1eqdsNsv78h4iu/+n42pIZ3sqZLUlTe3PvIcLOeytHTC4K0bOETCm4BowXcKLv+H4sb2iIP4/ImxLIYEO1jsBwj5723WkYXvZmDO+2lx+Zsk/B/tf0vcZBqfmvqQ9MVlfal4kdpoJnFlxOGXFkX6aZT//LMeuU0lE60WKkSnpPaUD0ETVjSdvBb/+HrkgUZKN+w4aG6hrYusSUdrdwxxT/TPrTQkHyK3LmdJCzvj1yRVPgre8o/dImN4I11DSiC708h7l3cxqGVb3TiB8PreL8/z3p/axnfsygA7hrwAOOcZUZevwQBxJL5icnwoK0Zep6biDmt+O/yBWJfsFk6fY+6flXz4WUe2ayRRohaJvXmSj5qHKypLJWQtzTidtSL+efs2CYrMLdIFeoAheCuDk8trJEqKHfnbcwXD9tOzBuDzRluvn5zsUEuifHxs6L1YOyclHoC+nvaS7CTYbWlbbAw4y1t+htpY8KshDXCC6QO8Kq3jUnsvxhpJqM262XhZUak2dhMD2nssD2sMNlyl/36A==
1. Why would a GWAS using a larger number of SNPs be more valuable than a study utilizing fewer SNPs?
Question
2.2
65YDeuHWOsCvPqQuaeJJbgKLtaYT5566mmvym9j4IQ+LTXW4TYqdTyYs3g2id7bnQ2/YOCTiJVKIe8BiW86/zwLZO0mp8CkS432FSiLKygINqSMap7HxQZMIQjgAikYJD40vjo2U24kgjPiNbHaF6gLwZm3J0tsb9HaV07zrLC2F3OPYYwAcD0zL/ZguQTnHJ44fclqn1FDVwZ9hgjL0r5NQnCPhUp88pznJEaT3vmKU/veLaO8LOXG5o1xE7cNu7C/bsJC9tzP9z/sQ43BeMfiNqfN7aKyuooo0aCTGL933WR0OxTQcKI/gfh1CkbaTWxAhb4SxzOMZybddVEQHOiZ2uxKQwOVec7MhkIQXSr58IAuOovluPEzQSqdlBCNCWAh4moDdSUM62voApvwwbA2pjBjhxlsJ666+GFWbKAjLy6yTuB7yvLVZ9p2gAothdzZDLl+c3psO5Tk7B6QbVncGcjaVrzqacj4zlSez3Y6CFTi99KfLLXb0tscJRTnZS2tRKic+XA2eOe233+gvKtGBtvLd2kWWUQ/aKlVS3XwKjjj0aTf+kuXe5Lg2hQVY3lTdvn7NDUAFj45v7HGq/09G5vrXh0FioQMICHRL01ukRrZ/LsPtYT2YMn0=
2. A GWAS identified the complement factor H (CFH) gene as associated with age-related macular degeneration (AMD). Would you expect a person with wild-type CFH to develop AMD? Why or why not?
Question
2.3
kaflQwBcAV0nHHDsQPhwcjDlMUrDgFxUyH8MpG9m7+CveJC8bYxxtCaDfo+/vgdQRtKIWyAPDDRfSlQaP/PBT3qPJKF8KsKc0DnymEJGQ5i8yplN72oPt0GoMETM554X9a0gciLEJUDpMshBGKXUPHJqhqvutXg2WFXiDzWL6eWIhNcDHDNA+poKnuYb20oeOEQ1NHePcETd5gPXONOU3Ml+s3m2xMly+2eU9gzbyZglEJT6Ljkw4Mt40/J/Jt7c04aTlLEEJ6X5yMdvqjQFVrzeP37KB1P67QhhfCHsSzyJ+8uuEXPwt1uFJFRvFfqgDHlkfgBsuV3tdOxkfeE767P9b3djEbqUc0xkaQppq+13/MQzlebA1SMPDBuXbg/Jb5Wa8uQpf9Q9rQbNLvXZ14QAC/1gwakKMetL5dvHPVc5CQa8UgE6yrJ3TVZhwHikuzIErC39JfJ/59Y6S6yZ2m6tTOorFn5OM46AfYqcQbvJMQYShHQM0ScMedf5vNuFjDF/XaA+dz8Gr4Wvsnp0IxmrX6+FDXYo
3. In what way was the GWAS of age-related macular degeneration (AMD) particularly helpful?
Question
2.4
taRHo7GRZWKmiiXJ4WzCDI+14mxg+U/yJdPjtrOgSjdbuj7xqq5BT+1tx55P5dbpRgVUSIk3k2kANtlQwF9VpH5u5wrKlEnCzGokNkCjgWz5hRUZCLzveTQuEiqLVGsq9DGv86Ap4iy9Cvsv8VUQ2N7qAsc/OILwsZhR/Wdndjcj0DHQ6LHosTRExWjAWWQ6v9kkhm9lBM7YZmfz13+h9lCw0R2nsh9PbQZTUkmon12SXr6GfUUpkG8G1+J64klOoOyY0m9x45ahhO6E4xp1/XUB4hrzjn2aRLm1vq8j+iQOxxhl0kNkNtl1bN3CmFaPwbwm/fIIGQ1mNgH/MDjtTXF0RWKgQxgmP3OMxWjwVa/nqmxoCD93xpHXZ2vJA7DoLVx60a8BJkG+DgTK9l2jWhue+LvRGGRyxvDDuVywAYj83YB5xv2pc8LyGZbN9zVnFGv5YpB58urptX4olS7dYX7txUw1HndRsYv+ZDOzxoWJ9K9TTrMiKl3PTcBHY29GkqwdRJF5dt1IXjVyH1iEZLBXYzLGCkC5tNG9a3HTCWetfSydKkWJnf9tiepswq8+rMUVFCFOa6MhDVXTpIDq7BoJvXbiRZ05geEXLMA4fKv+Pdz26zvm6WnJ37rDVSSO+KzKnevPbGWjIMF3WXX7va37Nc21gtxd68AIMbAMCahWBqdk/dHDc0GKfxHm6UX3HD3mqA==
4. Personalized medicine strives to treat individual patients best according to the information available about that patient. How can results from a GWAS aid the field of personalized medicine?
Question
2.5
aTwobFjPHIT/0FxQ3pAqG062H+rG3Vr71s3eTnrxlnalj9DBI+52HsMRbeDZBqM9QFYwbk0llyTTsARhhnDM6qLogDTeRB5n8JDm+prrTzfHUFG/3rV4L/RQhv4/sYmK8nJbOPQVMM/9deu0Z9elH5uJ5VgbZkbffmUG9pBXy/taEfuPVyQXWIlu55ez0sTcbJrklOl8Gyc9vW3twikCnaVmiF4hWJL02sftT7hvTFr0WfKXN+//cL/xKgFBaA92xwe//V/D3BqOk7CUSyfM2S121oXP14b6Jv9McbeZDO/nM2Ml88ZmxKBY+RIk1FA1Y+7KUb4Froh4U+/tNyFwcIjWY4Cr25s3jQdVzgxdqht7Piyr/AwMOZMDLUXVCJXIURa9xEPtdgt63zG6H81U/e41O/9muxtoE+11YkdMNp8VfizwJ0zLauHslBaPe/8IRecxFA==
5. Which of the following resources is NOT required to perform a GWAS?